Data-Driven Decisions in Competitive Markets
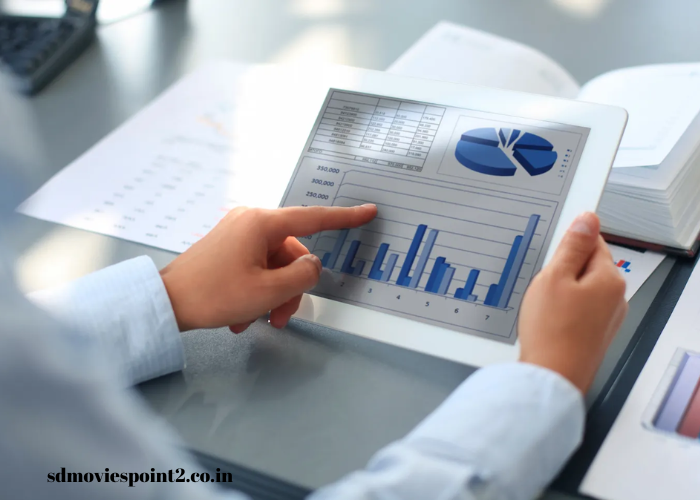
In today’s rapidly evolving business environment, the role of data has become increasingly critical in shaping the strategies and decisions of organizations. The advent of big data, advanced analytics, and artificial intelligence has revolutionized how businesses operate, particularly in competitive markets where the margin for error is slim. Companies that harness the power of data to make informed decisions are more likely to thrive, while those that rely on intuition or outdated methods may find themselves lagging behind. This article delves into the significance of data-driven decisions in competitive markets, the methodologies involved, the challenges faced, and the future outlook for businesses that prioritize data in their decision-making processes.
The Importance of Data-Driven Decisions
In a competitive market, every decision can have a significant impact on a company’s bottom line. Whether it’s entering a new market, launching a new product, or optimizing a supply chain, the ability to make informed decisions based on accurate and timely data is crucial. Data-driven decisions enable organizations to:
Enhance Efficiency: By analyzing data, businesses can identify inefficiencies in their processes and make adjustments to streamline operations. For example, data analysis can reveal bottlenecks in production or supply chain delays, allowing companies to address these issues promptly.
Improve Customer Satisfaction: Understanding customer behavior through data analysis helps businesses tailor their products and services to meet customer needs. This can lead to increased customer satisfaction and loyalty, which are critical in a competitive market.
Mitigate Risks: Data-driven decisions allow companies to assess potential risks and take proactive measures to mitigate them. For instance, predictive analytics can forecast market trends, enabling businesses to prepare for potential downturns or capitalize on emerging opportunities.
Optimize Marketing Strategies: Data analytics enables businesses to segment their target audience more effectively, personalize marketing campaigns, and measure the success of different strategies. This leads to more efficient allocation of marketing resources and higher returns on investment.
Drive Innovation: By analyzing market trends and customer feedback, companies can identify unmet needs and develop innovative products or services that differentiate them from competitors.
Methodologies in Data-Driven Decision Making
The process of making data-driven decisions involves several methodologies, each with its own set of tools and techniques. The following are some of the key methodologies used in data-driven decision-making:
Descriptive Analytics: This methodology focuses on understanding past performance by analyzing historical data. Descriptive analytics provides insights into what has happened in the business, allowing companies to learn from past successes and failures.
Predictive Analytics: Predictive analytics uses statistical models and machine learning algorithms to forecast future outcomes based on historical data. Businesses use predictive analytics to anticipate market trends, customer behavior, and potential risks, allowing them to make proactive decisions.
Prescriptive Analytics: This advanced methodology goes beyond prediction by recommending specific actions to achieve desired outcomes. Prescriptive analytics combines data, algorithms, and business rules to suggest the best course of action in a given situation.
Real-Time Analytics: In today’s fast-paced market, real-time analytics has become increasingly important. This methodology involves analyzing data as it is generated, allowing businesses to make decisions on the fly. Real-time analytics is particularly valuable in industries like finance, where market conditions can change rapidly.
A/B Testing: This methodology involves comparing two or more versions of a product, service, or marketing campaign to determine which performs better. A/B testing allows businesses to experiment with different strategies and make data-driven decisions based on the results.
Challenges in Implementing Data-Driven Decisions
While the benefits of data-driven decision-making are clear, implementing this approach is not without its challenges. Some of the key challenges businesses face include:
Data Quality: The accuracy and reliability of data are critical for making informed decisions. However, many businesses struggle with data quality issues, such as incomplete or outdated data, which can lead to incorrect conclusions and poor decision-making.
Data Integration: In many organizations, data is stored in silos across different departments, making it difficult to integrate and analyze data from multiple sources. Data integration is essential for gaining a holistic view of the business and making informed decisions.
Data Security and Privacy: With the increasing amount of data being collected and analyzed, businesses must ensure that they are protecting sensitive information and complying with data privacy regulations. Data breaches and privacy violations can have severe consequences, including legal penalties and damage to a company’s reputation.
Skill Gaps: Data-driven decision-making requires specialized skills in data analysis, machine learning, and data visualization. However, many organizations lack the necessary talent to fully leverage their data. Closing this skill gap is essential for businesses that want to remain competitive.
Cultural Resistance: Transitioning to a data-driven approach can be challenging for organizations with a traditional decision-making culture. Employees and leaders who are accustomed to making decisions based on intuition or experience may resist the shift to data-driven decision-making.
The Future of Data-Driven Decisions in Competitive Markets
As the business landscape continues to evolve, the importance of data-driven decisions is only expected to grow. Several trends are shaping the future of data-driven decision-making in competitive markets:
Increased Adoption of AI and Machine Learning: Artificial intelligence and machine learning are transforming the way businesses analyze data and make decisions. These technologies enable organizations to process vast amounts of data quickly and uncover insights that were previously impossible to detect. As AI and machine learning continue to advance, their adoption in data-driven decision-making will likely become more widespread.
Expansion of Data Sources: The proliferation of the Internet of Things (IoT), social media, and mobile devices is generating an unprecedented amount of data. Businesses that can harness and analyze this data will have a competitive edge in understanding market trends and customer behavior.
Emphasis on Data Ethics: As data collection and analysis become more pervasive, there will be a growing emphasis on data ethics. Businesses will need to ensure that they are using data responsibly and transparently, respecting customer privacy, and avoiding biases in their algorithms.
Integration of Data-Driven Culture: For data-driven decision-making to be truly effective, it must be ingrained in the company culture. This means fostering a mindset that values data and encourages employees at all levels to use data in their decision-making processes.
Collaboration Between Humans and Machines: The future of data-driven decisions will likely involve a collaborative approach where humans and machines work together. While machines can process data and generate insights, human judgment and intuition will still play a critical role in interpreting the results and making final decisions.
Conclusion
In conclusion, data-driven decisions are becoming increasingly essential in competitive markets where businesses must constantly adapt to changing conditions and make informed decisions quickly. By leveraging data analytics, companies can enhance efficiency, improve customer satisfaction, mitigate risks, optimize marketing strategies, and drive innovation. However, implementing data-driven decision-making is not without its challenges, including data quality, integration, security, skill gaps, and cultural resistance.
As technology continues to evolve, the future of data-driven decisions will be shaped by the adoption of AI and machine learning, the expansion of data sources, a focus on data ethics, the integration of a data-driven culture, and the collaboration between humans and machines. Businesses that prioritize data in their decision-making processes will be better positioned to succeed in competitive markets.
Leave a Comment